Introduction
Dementia is one of the leading causes of disability and dependency of elderly people. It is a neurodegenerative, fatal disease of chronic or progressive nature. It typically affects memory, thinking, orientation, behaviour and the ability to perform everyday activities. iHomeLab aims at developing a novel cognitive impairment screening test (CIST) to support the diagnosis of a human being’s cognitive and mental state. Mild cognitive impairment (MCI), an early stage of dementia, is often not diagnosed by primary care physicians. As cognitive impairment and deficiencies are associated with decreased quality of life (Lawson et al., 2014), preliminary evaluation and screening of cognitive deficits and subsequent rehabilitation methods could lead to better quality of life.
Methods
CIST is a system to estimate patients› cognitive state by evaluating patients› pupillary response during a cognitive test. It is made up of two main components, a portable and wearable eye-tracker system and CIST software. The CIST software is developed in Python and is made up of the three sections to set up the eye-tracker system, run an experiment and evaluate patients› cognitive state. The CIST test comprises of a visual-based digit span test based on the Wechsler Memory 70 Scale-III Memory Test. This test invokes a pupillary response on the person conducting the test. CIST analyses patients› pupillary response and estimates the cognitive state based on a machine learning algorithm and trained support vector machine (SVM) model.
Discussion
An initial report of the classification was created. The results indicate a particularly high precision rate of 100%. The reason for this is that the classifier is trained on optimised and distinct cognitive normal (CN) and cognitive impaired (CI) demo data. Demo datasets were used due to the outbreak of the SARS-CoV-2 virus, which prevented the recording of realistic data from CN and CI patients. Unfortunately, there were no publically available equivalent datasets available. Two demo datasets were created on the basis of one realistic dataset. The CI demo datasets were computed based on a statement from Kremen et al., 2019, that patients with MCI have reduced pupillary responses resulting in a flatter pupil dilation curve. Recording realistic CN and CI is crucial for CIST to accurately estimate patients› cognitive state, and is a possible continuation of this work.
Conclusion
This thesis provides an initial concept for CIST. Currently, the classifier is trained on artificially created datasets. In future projects, recording real ocular data from CN and CI patients will be important to verify the classification. An equal number of CN and CI patients would be required to comply with best practices in clinical evaluation. This system could be potentially applied to estimate other diseases and conditions, such as Parkinson’s, ADHD and autism by training the classifier on the eye movement data acquired from the appropriate patients.
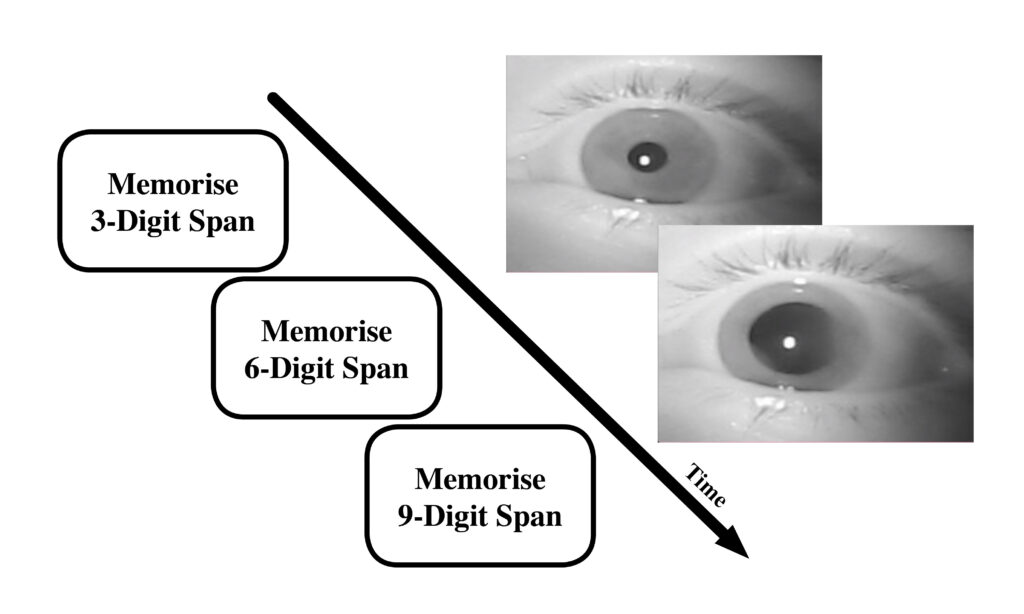
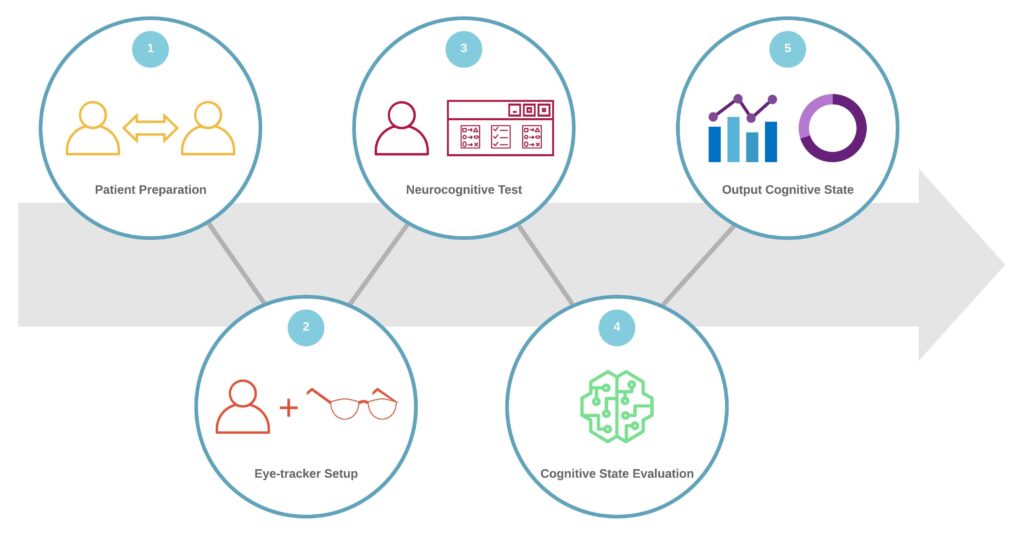
Figure 3: Cognitive Impairment Screening Test (CIST) workflow.
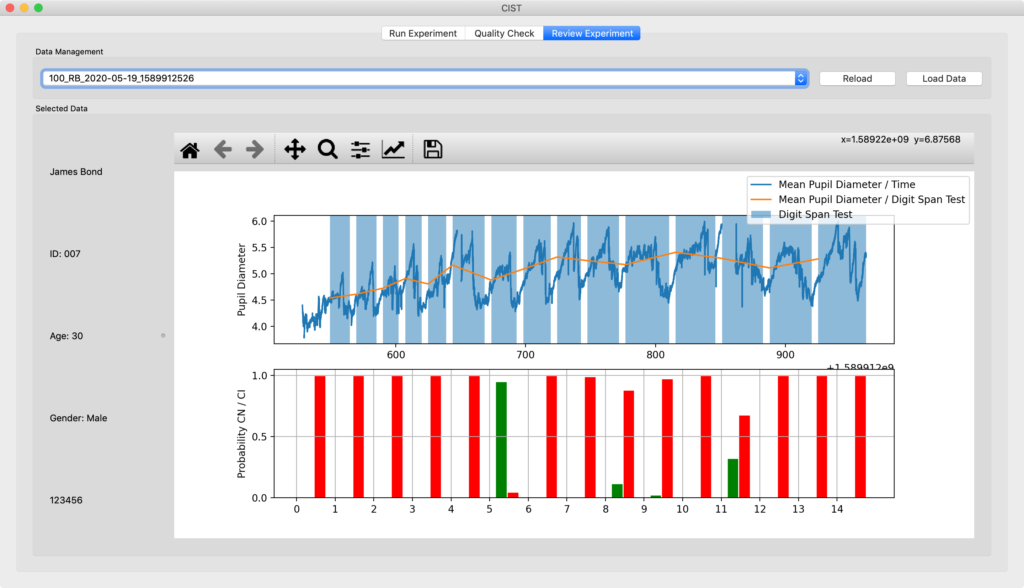